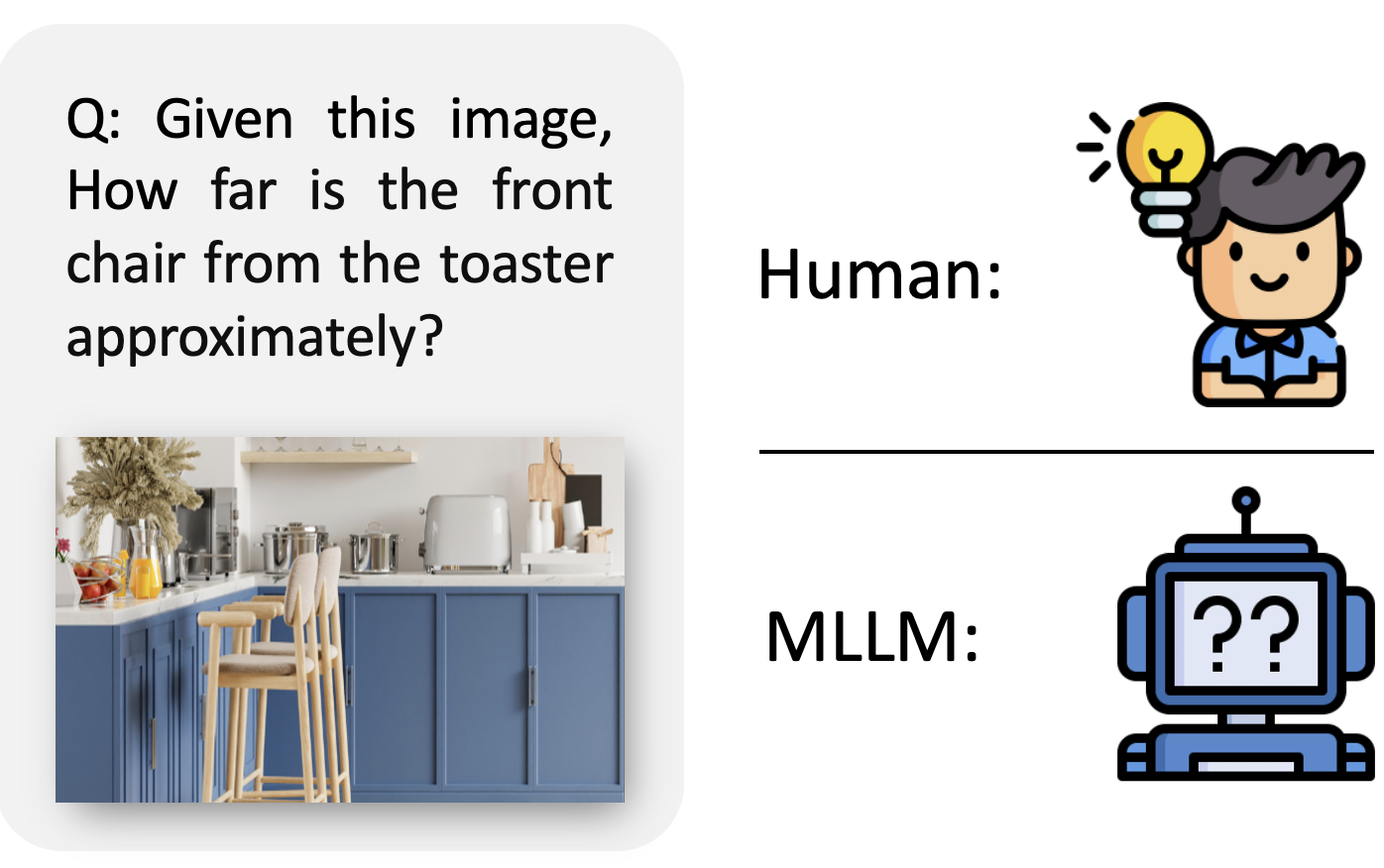
SpatialVLM is a product of Google DeepMind, designed to understand and reason about spatial relationships natively. The motivation behind its creation lies in the human ability to effortlessly determine spatial relationships, such as the positioning of objects relative to each other or estimating distances and sizes. This natural proficiency in direct spatial reasoning tasks contrasts with the current limitations of Vision-Language Models (VLMs).
VLMs have demonstrated remarkable performance in certain Visual Question Answering (VQA) tasks, but they still lack capabilities in 3D spatial reasoning, such as recognizing quantitative relationships of physical objects like distances or sizes. The SpatialVLM project hypothesizes that the limited spatial reasoning abilities of current VLMs are due to the lack of 3D spatial data during training. To address this, the team developed an automatic 3D spatial VQA data generation framework that scales up to 2 billion VQA examples on 10 million real-world images.
The SpatialVLM project is a significant step forward in the field of AI, as it introduces the first internet-scale 3D spatial reasoning dataset in metric space. By training a VLM on such data, the team significantly enhanced its ability on both qualitative and quantitative spatial VQA. This development unlocks novel downstream applications in chain-of-thought spatial reasoning.
One of the key applications of SpatialVLM is in the field of robotics. For instance, Visual Language Maps (VLMaps) enable spatial goal navigation with language instructions. The key idea behind building a VLMap is to fuse pretrained visual-language features into a 3D map. This can be done by computing dense pixel-level embeddings from an existing visual-language model, such as SpatialVLM. This opens up future opportunities for robots to understand and navigate their environment more effectively.
Another application of SpatialVLM is in the field of reinforcement learning. Vision-Language Models can be used as zero-shot reward models (VLM-RMs) to specify tasks via text prompts. For example, a humanoid robot can be trained to learn complex tasks from a text description, such as "a humanoid robot kneeling". This suggests that future VLMs, endowed with spatial reasoning capabilities like SpatialVLM, will become more and more useful reward models for a wide range of reinforcement learning tasks.
In conclusion, Google's SpatialVLM is a pioneering development in the field of AI, bridging the gap between machine learning models and spatial reasoning. Its applications in robotics and reinforcement learning are just the tip of the iceberg, and as the technology matures, we can expect to see it being used in a wide range of industries and applications. The future of AI is spatial, and SpatialVLM is leading the way.